This course is certified by the European society for precision engineering & nanotechnology (euspen) and the Dutch Society for Precision Engineering (DSPE) and leads to the ECP2-certificate.
Do you also have a motion system that has the same error for each task? Have you also been inspired by the many recent successes of learning, and want to investigate what learning for your machine could imply? Or are you excited about advanced motion feedforward control that even includes the 4th derivative of the set point signal? This course enables you to improve the performance of your system by advanced feedforward and learning control by learning from data.
In recent years, classical feedback controllers and feedforward controllers have been further developed towards advanced feedforward. This includes the use of higher-order derivatives of the setpoint signal, including jerk, snap, etc. In addition, the use of input shapers and rational feedforward controllers allows an even better performance, where new techniques have been developed to calculate such signals.
In addition, a lot of new results have been obtained at the intersection of control and machine learning. Successful developments include techniques that are related to iterative learning control and repetitive control, which applies to industrial systems, including pick-and-place machines or batch processes that perform the same task over and over again. When exactly the same task is performed, disturbances act on the system identically over the tasks. Think, for instance, about a disturbance torque profile, from unbalance in an axis, or from unknown friction effects. The key idea is these learning control techniques can completely compensate for these disturbances, leading to a typical order of magnitude reduction of servo errors. These techniques can achieve perfect performance. In addition, in recent years, these have been further extended to learn the optimal parameters of advanced feedforward controllers, i.e., using higher-order derivatives, input shapers, and even rational feedforward controllers. In addition, major developments include the use of techniques from machine learning, including Gaussian Processes.
This new and extended course starts by recapitulating classical feedforward, and covers an in-depth treatment of iterative learning control, repetitive control, and new advanced feedforward approaches, some of which are at the intersection with machine learning techniques. The course covers:
- theory, e.g., understanding the convergence of learning control from classical feedback;
- design, learning how to design advanced feedforward and learning from typical motion control design approaches (loop-shaping);
- connections to new developments and being able to understand their relevance, including recent ideas from machine learning;
- algorithms, full coverage of tailor-made Matlab-algorithms (with possibility to take these home).
This training is available for open enrollment as well as for in-company sessions.
Objective
After attending this course, participants are enabled to:
- apply basic feedforward for motion control;
- recognize which technique is applicable to the specific application at hand (e.g., iterative learning control, repetitive control, and new advanced feed-forward algorithms);
- analyze and design ILC controllers addressing both performance and robustness (convergence), both in frequency domain and lifted domain;
- implement and analyze repetitive controllers;
- implement and analyze basis functions in ILC;
- auto tuning of advanced feedforward controllers;
- apply input shapers and rational feedforward;
- design and implement model-free learning controllers;
- able to read, understand, and implement recent developments in the field, including many ideas that relate to machine learning; and
- obtain hands-on experience on an industrial mechanical positioning system.
Target audience
This course is intended for engineers involved in motion systems who want to gain more insight into the possibilities in learning from data in machines, and implementations of advanced feedforward and learning control in an industrial setting.
It is recommended that participants already have a Bachelor or Master education in electrical engineering, mechanical engineering, mechatronics, physics, or equivalent practical experience and must have basic understanding of servo control.
This course is particularly suitable for engineers having followed the course in 'Motion control tuning', or who have obtained similar expertise through training or experience.
Program
The following topics are treated:
- Overview application areas;
- Iterative learning control: basic principles, frequency domain approach, convergence and robustness analysis, and design;
- Repetitive control: basic principles, theory, design, and algorithms;
- Lifted iterative learning control: basic principles, analysis, optimal design;
- Basis functions in iterative learning control;
- Automated feedforward tuning;
- Input shaping and rational feedforward;
- Recent developments, including model-free learning controller design (IIC) and results relating to machine learning;
- Practical examples;
- Simulation and design of systems using Matlab and SIMULINK;
- Hands-on experience with real-time implementation using SIMULINK on HP printer setup.
Certification
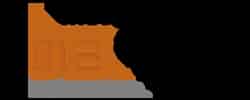

More information
Remarks from participants