In-depth lectures, step-by-step methodology, many examples and worked exercises on the theory and how to apply the tools.
A hands-on case study as guided team exercise serves to integrate the steps.
Training time is roughly 50/50 divided between theory and practice exercises, including group exercises.
Course material: theory reader with a copy of the slides, exercises, worked exercises in Excel and Minitab.
Applied statistics is a key enabling skill in Research and Development. From setting up the experiments with adequate statistical power in early research, to design exploration, optimization and tolerance analysis in development and engineering – all phases in R&D benefit from correct application of appropriate statistical tools.
In research and early development, measurement statistics are used to obtain the measurement error and subsequently calculate a sample size that large enough to obtain sufficient statistical power– the ability to see an effect if it is present. For example, using duplicate measurements, the power of finding a difference between two prototypes when the difference is about equal to the measurement error is below 10%. Underpowered experiments are undesirable because they result in a waste of time and money. Pursuing an underpowered experimental has no merit as it will not yield a repeatable result, instead either the measurement needs to be improved to reduce the error, or the sample size needs to increase, i.e., experiments have to be repeated more often.
In later development and in engineering, activities pursue fulfilling a function in terms of a specific output parameter ending up inside a required range. Failure rate is the proportion of instances where the output is outside of the desired specification window and the intended function is not fulfilled as required. A high failure rate is undesirable, it is a hallmark of bad quality and carries a financial penalty.
Part of the failure rate is ‘designed in’ – it is the result of a combination of design choices and design input variations, resulting in a distribution of the output parameter that partly falls outside the specification limits. Direct measurement of this failure rate to guide design decisions is typically not feasible as it needs too many tests – for example, a failure rate of 1% implies that on average 100 tests need to be performed to see 1 failure. To see the designed-in failure rate with accuracy, many more tests are needed, and this is generally infeasible. This poses a dilemma: By the time you have sufficient data on the failure rate, the time window for changes in the design has already closed.
The timing dilemma is not uncommon, and not limited to statistical parameters. The general solution is to use a stand-in and resort to modelling, using calculated values instead of measured data – CAD modelling, Circuit modelling, FEM modelling and CFD modelling are all examples where a model is used to assess a design before direct testing is possible. In the same way, statistical modelling is used to investigate statistical behaviour of a design when testing is not feasible. Statistical modelling is foundational to design optimization and robust design. Specific experimental designs are used to derive surrogate functions that model the expected output values as a function of design inputs. Next, the surrogate functions are used to optimize. Finally, the expected distribution of the output parameter and the resulting designed -in failure rate are obtained from the surrogate function and the distribution of the input parameters.
This training is designed to familiarize participants with relevant statistical tools and way of working using linked statistical tools in their daily jobs. The trainer is a certified Master Black Belt in Design for Six Sigma with 35+ years of industry experience in R&D. All statistics are demonstrated using Minitab (industry standard) and Excel. The training contains exercises on individual statistical tools and a group exercise practicing the way of working, using the tools in conjunction. The training focus is on correct application of appropriate statistical tools to achieve development goals: “You do not need to know the workings of the engine in order to drive a car from A to B”. The training applies to continuous numerical parameters and covers both physical experiments and virtual experiments like CFD or FEM simulations.
This training is available for open enrollments in Eindhoven as well as for in-company sessions all over the world. For in-company sessions, the training can be adapted to your situation and special needs.
Objective
After successful completion of this course, the participant is able to:
- Use statistical tools and procedures to establish random measurement error.
- Estimate sample size to set up an experiment with a desired power (true positive rate) and confidence (true negative rate).
- Set up and analyse Design of Experiments and/or Response Surfaces to obtain the surrogate functions linking design inputs and outputs.
- Use multiple response optimization to combine design input settings for an optimal design output.
- Perform Monte Carlo simulation to calculate the output distribution and the failure rate.
- Do so with Minitab and/or Excel.
Intended for
This course is intended for researchers, (potential) system architects, system engineers, senior designers, design engineers and project leaders. Some experience in system or product development, from any discipline related to continuous numerical parameters like mechanics, physics, mechatronics, optics, or electronics, with an affinity to modelling is preferred. Prior education: BSc. No prior statistics knowledge is needed. For the exercises, a laptop with Excel and/or Minitab is needed. Minitab offers a free trial period and is often already in use at quality departments.
Program
- Normal distribution, probability
- Sampling, Sampling distribution, Central Limit Theorem, Confidence Interval of the mean, T distribution
- Hypothesis testing, 1 sample T, 2 sample T
- Normal approximation to the binominal, CI of proportion, 1 sample p, 2 sample p
- Power and sample size
- ANOVA, pooled standard deviation
- Measurement System Analysis, Gage Repeatability and Reproducibility
- Modelling the mean: Sampling (DOE, RSM) and Fitting (Least Squares Regression )
- Multiple Response Optimization
- Modelling the variation: Monte Carlo Simulation
- Individual and Group exercises
Methods
Certification
Participants will receive a High Tech Institute course certificate for attending this statistical modelling training.
Video
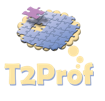